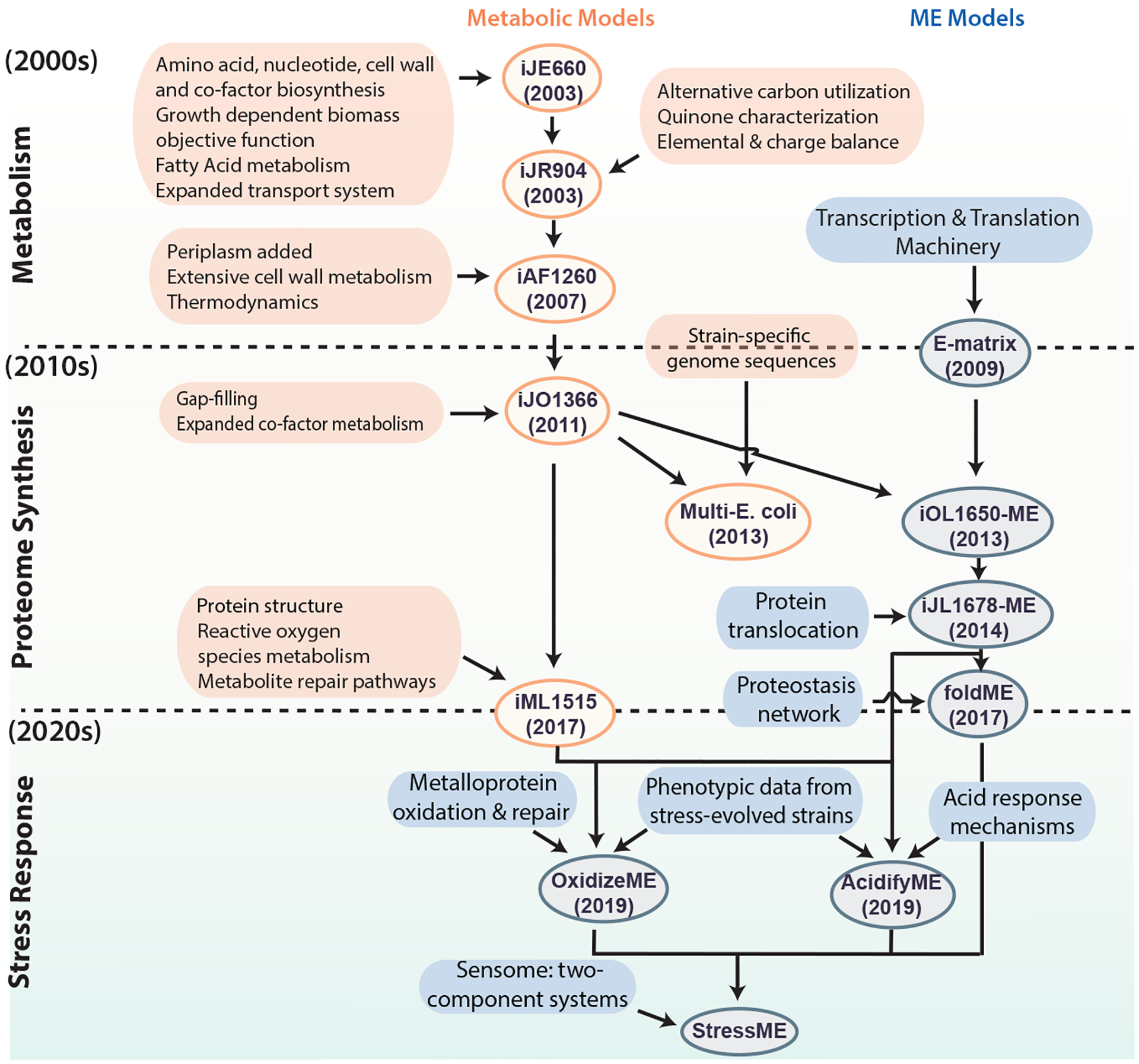
Genome-scale network reconstructions are built from curated and systematized knowledge that enables them to quantitatively describe genotype–phenotype relationships [1] [2]. In the case of metabolism, by mapping the annotated genome sequence to a metabolic knowledge base such as the Kyoto Encyclopedia of Genes and Genomes (KEGG) [3], one can reconstruct a metabolic network composed of all known metabolic reactions. Such genome-scale reconstructed networks represent organized and systematized knowledge-bases that have multiple uses, including conversion into computational models that interpret and predict phenotypic states and the consequences of environmental and genetic perturbations.
The metabolic network in a genome-scale metabolic reconstruction can be converted into a mathematical format — a stoichiometric matrix (S matrix) — where the columns represent reactions, rows represent metabolites, and each entry is the corresponding coefficient of a particular metabolite in a reaction. The resulting genome-scale model (GEM) is a mathematical representation of the reconstructed network that facilitates computation and prediction of multi-scale phenotypes through the optimization of an objective function of interest. These models have been developed for a wide variety of organisms, including iterative updates for critical organisms such as E. coli that continually improve the predictive performance of these models (Figure 1).
Flux balance analysis (FBA) is the most widely used approach to characterize GEMs [4]. GEMs can simulate metabolic flux states of the reconstructed network while incorporating multiple constraints to ensure the solution identified by FBA is physiologically relevant and compliant with governing constraints; such as the metabolic network topology represented by the S matrix, a steady-state assumption (for example, the internal metabolites must be produced and consumed in a flux-balanced manner), and other limits on nutrient uptake rates, enzyme capacities, and protein/gene expression profiles. The S matrix and the objective function define a system of linear equations that can be solved given the imposed constraints, resulting in a solution space (that is, a space where all feasible phenotypic states exist). FBA can identify a single or multiple optimal flux distributions that optimize the objective function in the solution space. FBA and many other GEM analysis methods are available through the COBRApy package in Python [5] or the COBRA Toolbox in MATLAB [6].
GEMs have been successfully implemented for a wide range of applications, including understanding microorganisms, metabolic engineering, drug development, prediction of enzyme functions, understanding microbial community interactions and human disease. These genome-scale models now enable us to develop pan-genome analyses [7] that provide mechanistic insights, detail the selection pressures on proteome allocation [8], and address stress phenotypes [9] [10].
Genome-scale metabolic modeling is continually being developed as a part of the Genome Design program managed by Dr. Daniel Zielinski, a Project Scientist in the Systems Biology Research Group. The Genome Design group is developing computational modeling and machine learning technologies to predict microbial phenotypes.
Citations/Further Reading
- Thiele I, Palsson BO. A protocol for generating a high-quality genome-scale metabolic reconstruction. Nat Protoc. 2010;5: 93–121.
- Norsigian CJ, Fang X, Seif Y, Monk JM, Palsson BO. A workflow for generating multi-strain genome-scale metabolic models of prokaryotes. Nat Protoc. 2020;15: 1–14.
- Kanehisa M, Furumichi M, Tanabe M, Sato Y, Morishima K. KEGG: new perspectives on genomes, pathways, diseases and drugs. Nucleic Acids Res. 2017;45: D353–D361.
- Orth JD, Thiele I, Palsson BØ. What is flux balance analysis? Nat Biotechnol. 2010;28: 245–248.
- Ebrahim A, Lerman JA, Palsson BO, Hyduke DR. COBRApy: COnstraints-Based Reconstruction and Analysis for Python. BMC Syst Biol. 2013;7: 74.
- Heirendt L, Arreckx S, Pfau T, Mendoza SN, Richelle A, Heinken A, et al. Creation and analysis of biochemical constraint-based models using the COBRA Toolbox v.3.0. Nat Protoc. 2019;14: 639–702.
- Monk JM, Charusanti P, Aziz RK, Lerman JA, Premyodhin N, Orth JD, et al. Genome-scale metabolic reconstructions of multiple Escherichia coli strains highlight strain-specific adaptations to nutritional environments. Proc Natl Acad Sci U S A. 2013;110: 20338–20343.
- Lloyd CJ, Ebrahim A, Yang L, King ZA, Catoiu E, O’Brien EJ, et al. COBRAme: A computational framework for genome-scale models of metabolism and gene expression. PLoS Comput Biol. 2018;14: e1006302.
- Du B, Olson CA, Sastry AV, Fang X, Phaneuf PV, Chen K, et al. Adaptive laboratory evolution of Escherichia coli under acid stress. Microbiology. 2020;166: 141–148.
- Chen K, Gao Y, Mih N, O’Brien EJ, Yang L, Palsson BO. Thermosensitivity of growth is determined by chaperone-mediated proteome reallocation. Proc Natl Acad Sci U S A. 2017;114: 11548–11553.
- Fang X, Lloyd CJ, Palsson BO. Reconstructing organisms in silico: genome-scale models and their emerging applications. Nat Rev Microbiol. 2020;18: 731–743.